Navigating the Promise and Perils of AI in Hiring: A Deep Dive into Data Bias and How to Mitigate It
By AI Enthusiast
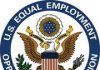
Artificial Intelligence (AI) has rapidly transformed many aspects of our lives, and hiring is no exception. From writing job descriptions to automating interviews, AI-powered tools promise to streamline HR processes and make hiring more efficient. But alongside these advantages lies a significant risk: data bias. If not addressed properly, AI could perpetuate or even amplify discrimination on an unprecedented scale.
AI in Hiring: A Double-Edged Sword
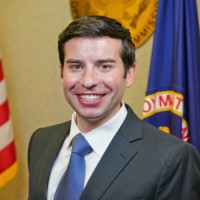
Keith Sonderling, Commissioner with the US Equal Opportunity Commission, recently emphasized the dual nature of AI in hiring during the AI World Government event. As he described, “The thought that AI would become mainstream in HR departments was closer to science fiction two years ago, but the pandemic has accelerated the rate at which AI is being used by employers.” Virtual recruiting has become the new norm, and AI plays a critical role in managing this shift.
The Great Resignation Meets AI
The labor market is currently undergoing a massive upheaval known as “the great resignation,” leading to a subsequent “great rehiring.” Sonderling noted, “AI will play a role in this like we have not seen before.” AI has already been in use for various HR tasks, such as chatting with applicants, predicting job acceptance likelihood, and even mapping out upskilling opportunities.
The Dark Side: Data Bias
While AI offers many advantages, its potential shortcomings cannot be ignored. “Carelessly implemented, AI could discriminate on a scale we have never seen before by an HR professional,” Sonderling warned. One of the primary culprits? Data bias.
AI models depend on training data, and if this data reflects existing biases in the workforce, those biases could be perpetuated. For instance, if a company’s current workforce is predominantly one race or gender, the AI could replicate that demographic in its hiring recommendations.
Real-World Examples
Amazon’s Hiring Algorithms
Amazon’s attempt to build a hiring application in 2014 is a cautionary tale. The AI model, trained on 10 years of the company’s hiring data, began to show a preference for male candidates. Despite efforts to correct it, the system was ultimately scrapped in 2017.
Facebook’s PERM Program
In another example, Facebook recently agreed to pay $14.25 million to settle claims that it violated federal recruitment rules and discriminated against American workers. This case underscores the importance of vigilance in AI’s application in hiring processes.
Mitigating AI Bias: Best Practices
To leverage AI’s potential while avoiding its pitfalls, companies must take proactive steps to mitigate data bias. Here are several strategies recommended by experts:
- **Diverse Training Datasets:** Ensure that training datasets reflect a broad spectrum of demographics to prevent the AI from replicating existing biases.
- **Ongoing Audits:** Regularly audit AI systems to identify and correct biases as new data becomes available.
- **Ethical Oversight:** Implement governance structures to oversee AI deployment and ensure ethical considerations are met.
- **Vendor Vetting:** Choose AI vendors who prioritize bias mitigation and diversity in their algorithms.
Example: HireVue
HireVue, a hiring platform from South Jordan, Utah, is designed to mitigate unfair hiring practices. “We actively work to prevent the introduction or propagation of bias against any group or individual,” states HireVue. They rigorously review their datasets and continuously improve their tools to promote diversity and equal opportunity.
Beyond Hiring: AI in Different Sectors
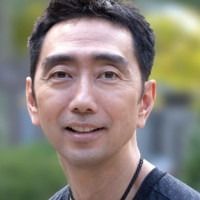
The issue of bias extends beyond hiring. According to Dr. Ed Ikeguchi, CEO of AiCure, “AI is only as strong as the data it’s fed,” highlighting the need for diverse and credible datasets across various industries.
For example, in healthcare, biased datasets can lead to inaccurate diagnoses and treatments, disproportionately affecting minority populations. This illustrates the broader implications of AI bias and the importance of a comprehensive approach to mitigating it.
Key Takeaways
While AI has the potential to revolutionize hiring and many other sectors, it also poses significant risks if not carefully managed. Here are the key points to remember:
- Understand the double-edged nature of AI: While AI can streamline hiring processes, it can also perpetuate significant biases.
- Regular audits and ethical oversight are crucial: Continuous monitoring and ethical governance can help mitigate AI’s negative impacts.
- Diverse datasets are essential: Training AI on diverse datasets can help prevent the replication of existing biases.
- Learn from real-world examples: Companies like Amazon and Facebook provide valuable lessons on the pitfalls of biased AI.