How Engineering Accountability is Transforming Government AI Practices
By John P. Desmond, AI Trends Editor
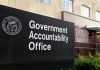
AI accountability is not just a trendy buzzword; it’s the backbone of trustworthy and ethical AI implementation within government systems. At the forefront of this movement are individuals like Taka Ariga and Bryce Goodman, whose efforts and frameworks are setting a gold standard for AI practices in federal agencies.
The Visionaries Leading the Charge
Introducing Taka Ariga
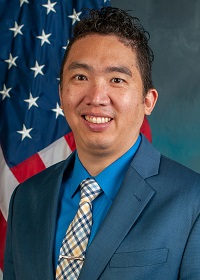
Taka Ariga, the chief data scientist and director at the US Government Accountability Office (GAO), has been a pioneer in developing an accountability framework specifically designed for AI applications. His work ensures that AI systems within government agencies are not just effective but also transparent, responsible, and equitable.
Insights from Bryce Goodman
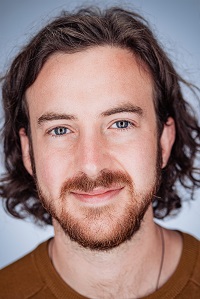
Bryce Goodman, chief strategist for AI and machine learning at the Defense Innovation Unit (DIU), has contributed significantly to establishing ethical guidelines that ensure responsible AI development within the defense sector. His multidisciplinary approach draws from his experiences in academia, military applications, and consultancy.
Building an Accountable AI Framework
The GAO’s AI Accountability Framework
In September 2020, Taka Ariga and his team embarked on a mission to build a comprehensive AI accountability framework. This initiative aimed to bridge the gap between high-level ethical principles and the pragmatic, day-to-day operations of AI engineers. The resulting framework, first published in June, categorizes the AI lifecycle into four “pillars” — Governance, Data, Monitoring, and Performance.
Governance Pillar: Assesses the organizational structures set up to oversee AI initiatives, including the roles and responsibilities of a Chief AI Officer.
Data Pillar: Ensures that training data is evaluated for representativeness and effectiveness, and scrutinizes the ethical implications of data use.
Performance Pillar: Examines the societal impact and legal compliance of AI systems, such as adherence to the Civil Rights Act.
Monitoring Pillar: Emphasizes continuous assessment to prevent “model drift” and ensure the long-term reliability of AI algorithms.
“AI is not a technology you deploy and forget,” Ariga emphasizes, underscoring the importance of continuous monitoring and adjustment.
DIU’s Ethical Guidelines for AI
The DIU, under the guidance of Bryce Goodman, has implemented ethical principles categorized under Responsible, Equitable, Traceable, Reliable, and Governable AI. These principles guide AI projects from conception to deployment, ensuring they are aligned with ethical standards and practical requirements.
Practical Applications and Lessons Learned
Goodman’s experience spans various AI applications, from humanitarian assistance and disaster response to predictive maintenance and counter-disinformation campaigns. Here are some key takeaways from his work:
- Define the Task Clearly: Only pursue AI solutions if they offer a distinct advantage.
- Set Benchmarks: Establish clear metrics for success before the project begins.
- Data Ownership: Clarify data ownership and ensure data is ethically sourced and consented for use.
- Stakeholder Responsibility: Identify responsible stakeholders and mission-holders to ensure accountability.
- Rollback Mechanisms: Have a contingency plan to revert changes if issues arise.
“Metrics are key. Simply measuring accuracy might not be adequate,” Goodman advises, stressing the need for comprehensive evaluation criteria.
A Unified Approach for AI Accountability
Both the GAO and DIU frameworks highlight the necessity of collaboration across government and commercial entities. The shared goal is to create an AI ecosystem that minimizes risks while maximizing benefits. These efforts are not about achieving perfection but avoiding catastrophic outcomes.
Ultimately, the aim is to make high-level ethical principles actionable and relatable for AI practitioners. As Goodman aptly puts it, “AI is not magic. It will not solve everything. It should only be used when necessary and only when it can provide a tangible advantage.”
Takeaways for AI Practitioners
For AI professionals inside and outside the government, these practices offer a roadmap not just for compliance but for excellence in ethical AI. They promote a balanced approach that values transparency, fairness, and ongoing accountability.
What frameworks or practices have you found helpful in your AI projects? Share your thoughts and experiences in the comments below.
Learn more about these initiatives at the AI World Government, the Government Accountability Office, the AI Accountability Framework, and the Defense Innovation Unit site.